The research areas of the Computational Physics & Data Science Research Group include aerodynamic thermal analysis and flow field analysis of aircraft, high-fidelity numerical computation and mechanism analysis of turbulence, as well as the application of data-driven methods such as artificial intelligence, modal reduction, and data assimilation in the field of fluid mechanics. Almost all flows in engineering practice and nature are turbulent (https://en.wikipedia.org/wiki/Turbulence), such as the flow field around aircraft, blood in the heart, turbulent rivers, sandstorms, atmospheric cyclones, and solar storms, with spatial scales ranging from millimeters to kilometers and temporal scales from milliseconds to decades. Precise measurement of turbulence at different scales encounters significant difficulties, thus, recreating and exploring turbulent flows of different scales in engineering practice and nature through high-performance computing methods in the laboratory is a challenging and exciting research endeavor. Additionally, over the past few years, we have witnessed how deep learning and data assimilation methods have profoundly changed every aspect of life. At the same time, how to apply deep learning and data assimilation methods to the field of fluid mechanics, leveraging powerful computing power and massive data to explore, predict, and control turbulence from new perspectives, is also an extremely important research direction.
We welcome students and scholars interested in the aforementioned research directions to visit and exchange ideas. Additionally, we welcome international students to apply for master's, doctoral, and postdoctoral positions in our research group. We will provide a harmonious and trusting work environment, and offer students ample professional guidance.
Representative Articles:
[J6] Zheng, Q., Li, T., Ma, B., Fu, L.*, & Li, X.* 2024 High-fidelity Reconstruction of Large-area Damaged Turbulent Fields with Physically-constrained Generative Adversarial Network. Physical Review Fluids, 9, 024608.
[J5] Zheng, Q., Yang, Y., Wang, J.* & Chen, S. 2022 Enstrophy production and flow topology in compressible isotropic turbulence with vibrational non-equilibrium. Journal of Fluid Mechanics,950, A21.
[J4] Zheng, Q., Wang, J.*, Alam, M. M., Noack, B. R., Li, H. & Chen, S. 2021 Transfer of internal energy fluctuation in compressible isotropic turbulence with vibrational nonequilibrium. Journal of Fluid Mechanics, 919, A26.
[J3] Zheng, Q., Wang, J.*, Noack, B. R., Li, H., Wan, M. & Chen, S. 2020 Vibrational relaxation in compressible isotropic turbulence with thermal non-equilibrium. Physical Review Fluids, 5, 044602.
[J2] Zheng, Q. & Alam, M. M.* 2019 Evolution of the wake of three inline square prisms. Physical Review Fluids, 4, 104701.
[J1] Zheng, Q. & Alam, M. M.* 2017 Intrinsic features of flow past three square prisms in side-by-side arrangement. Journal of Fluid Mechanics, 826, 996-1033.
Representative Results:
Flow Field and Aerodynamic-Thermal Analysis of Aircraft and Fluid Machinery
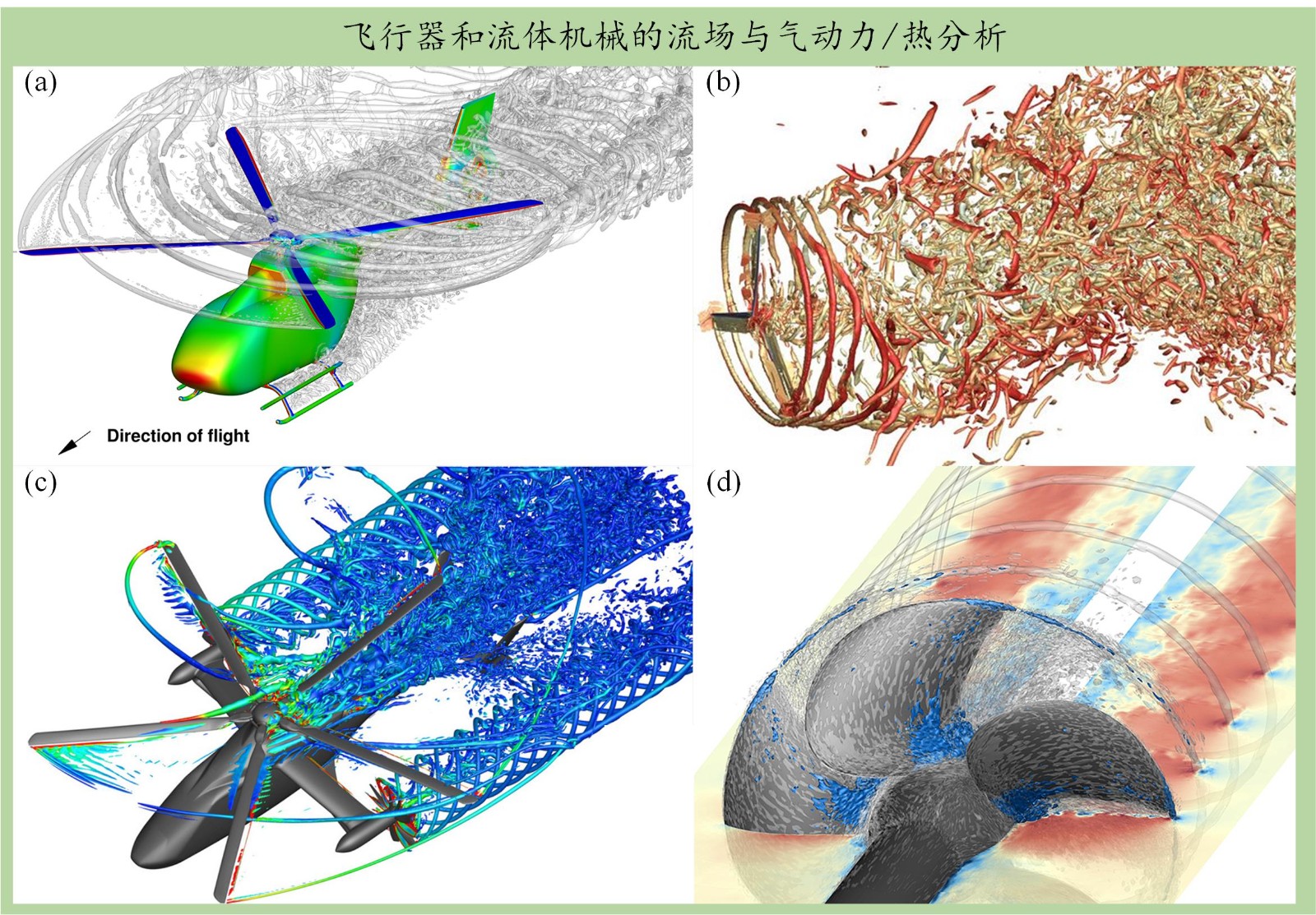
Application of Deep Learning Methods for Fluid Mechanics
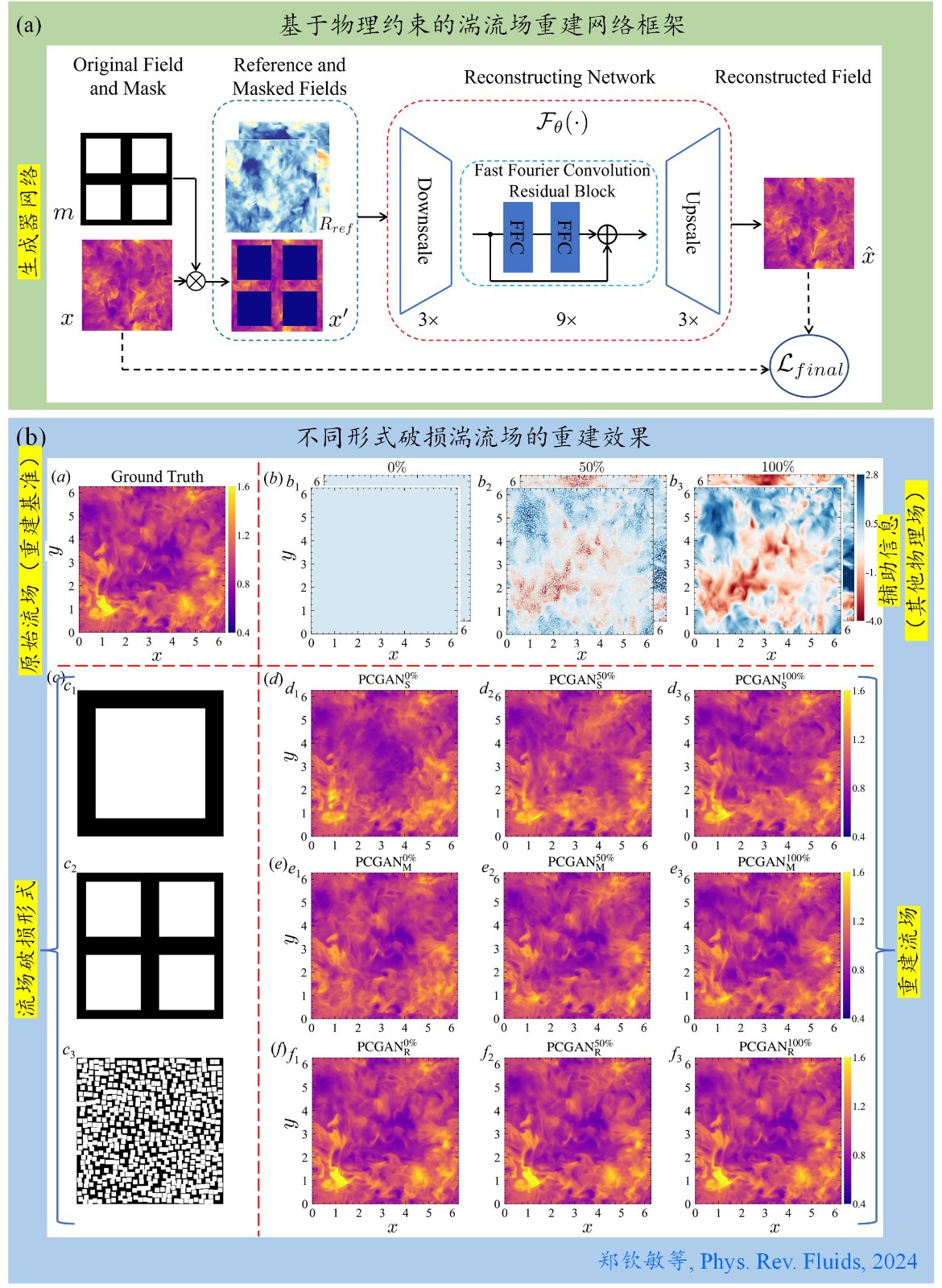
High-fidelity Numerical Simulation and Mechanism Analysis of Turbulence
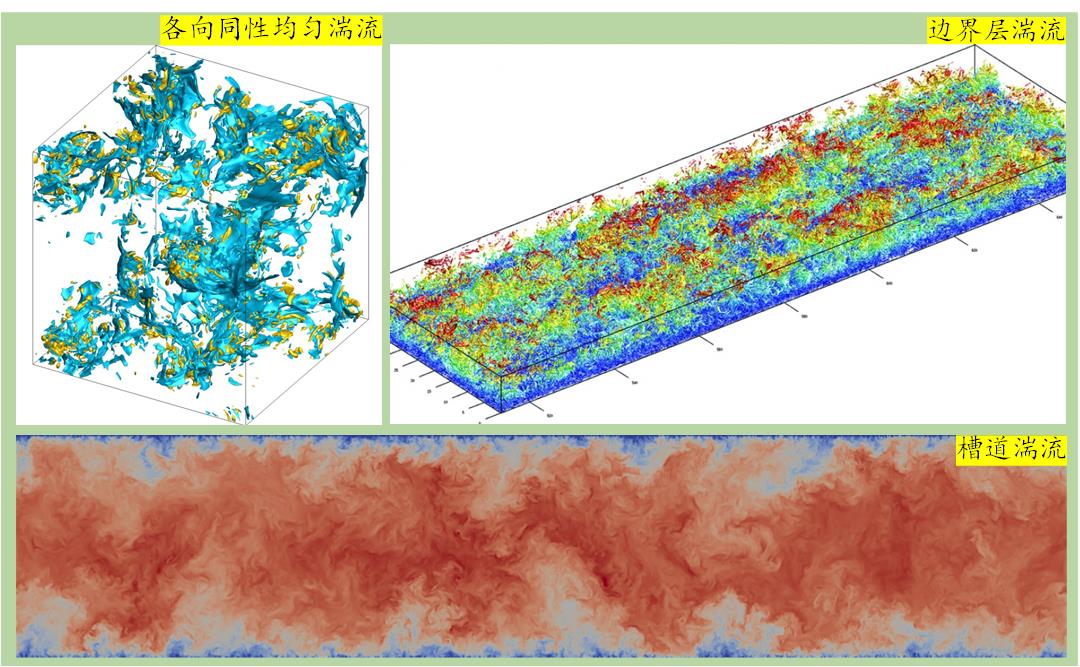
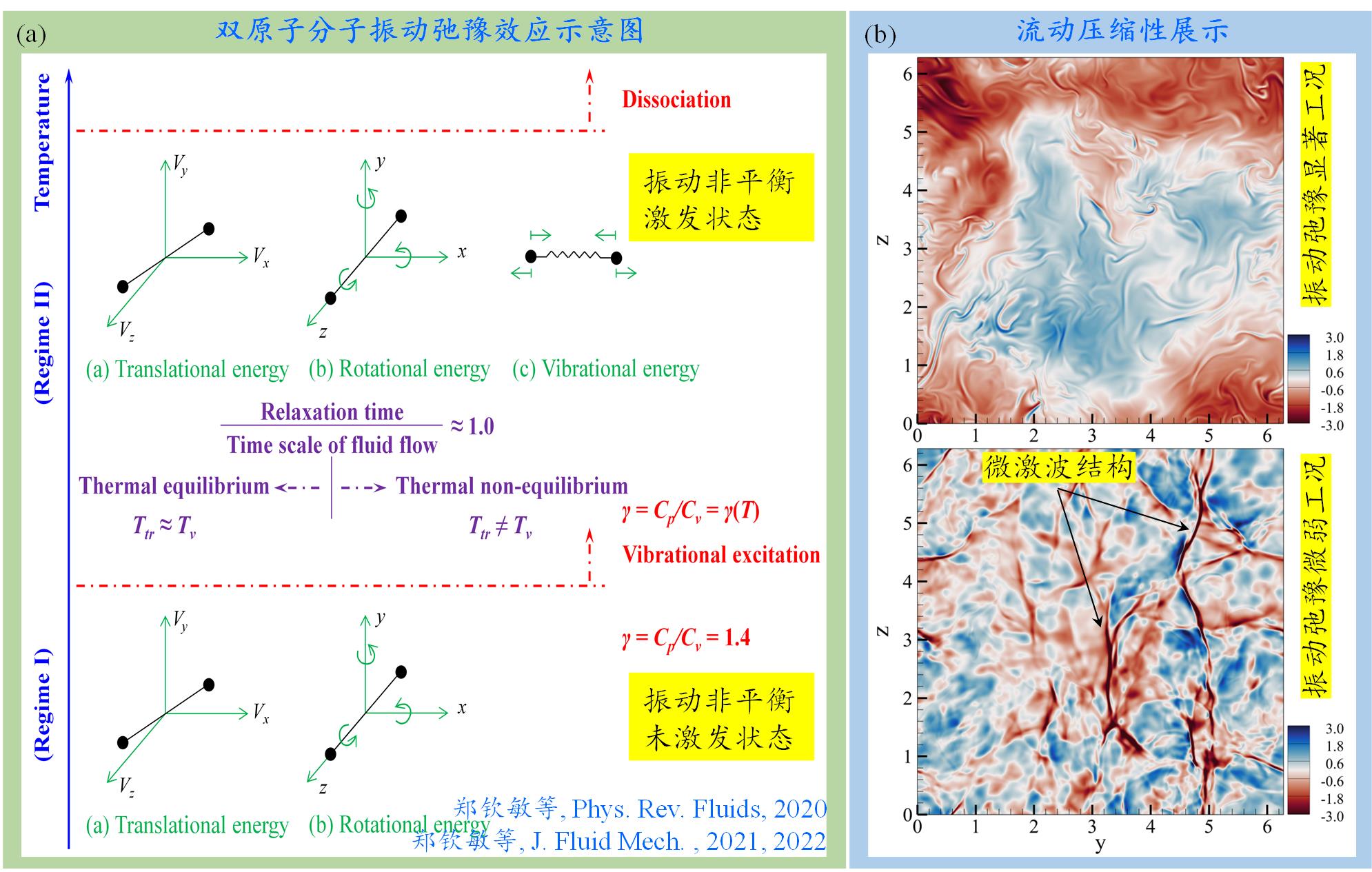
Application of Data-driven Methods for Fluid Mechanics
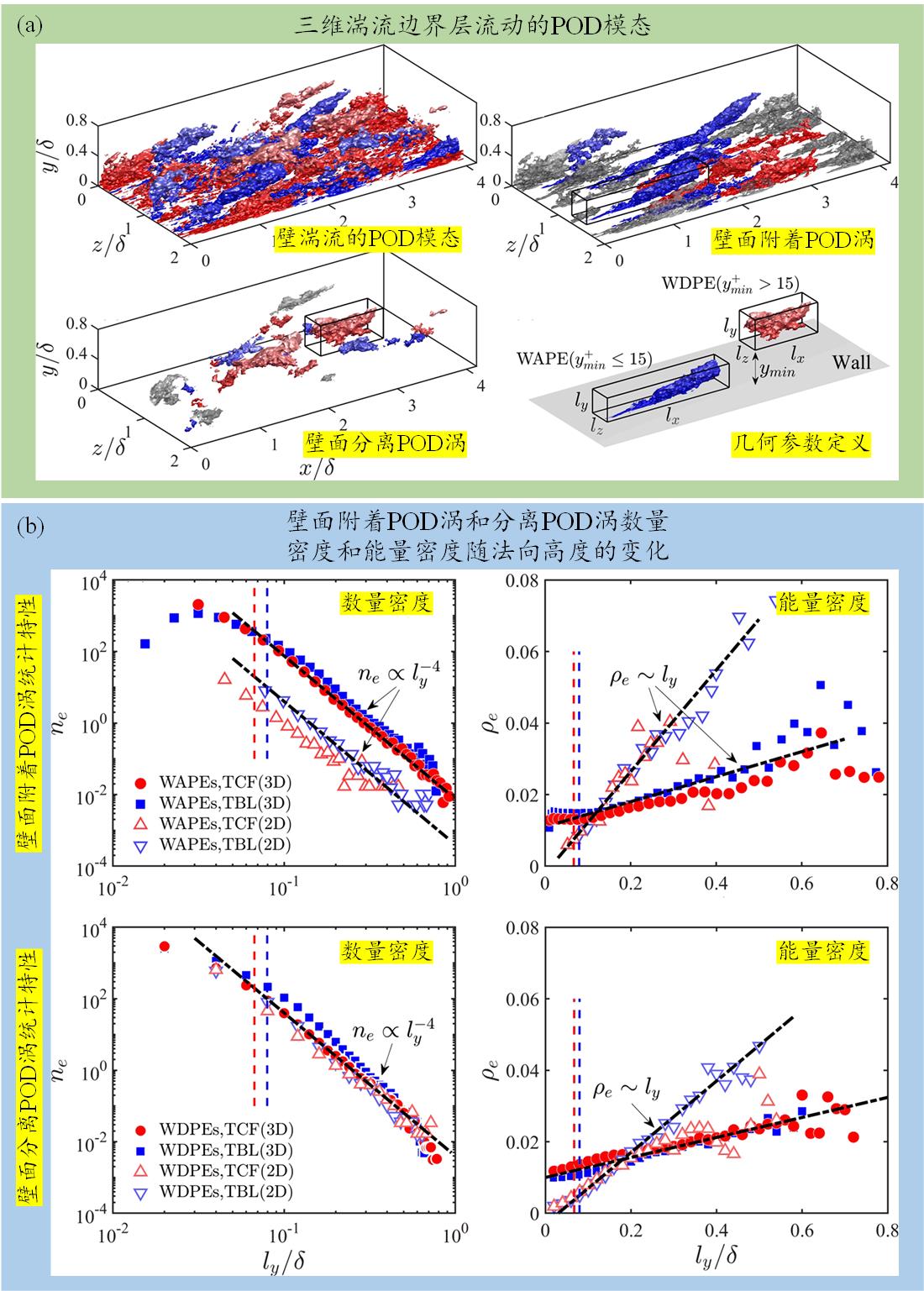